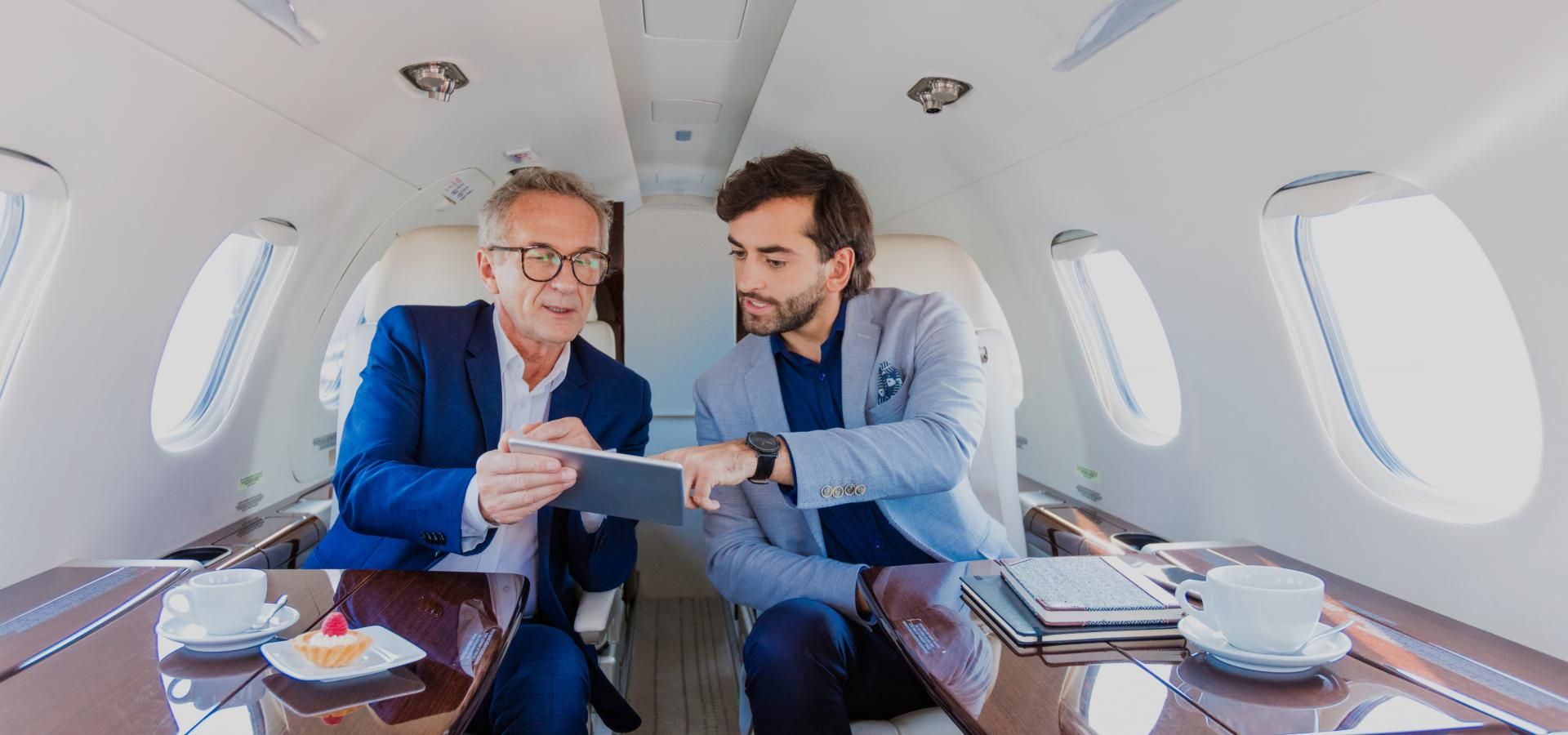
Artificial intelligence in wealth management: use cases, solutions & adoption guidelines
February 12, 2025
- Home
- Artificial intelligence
- AI in wealth management
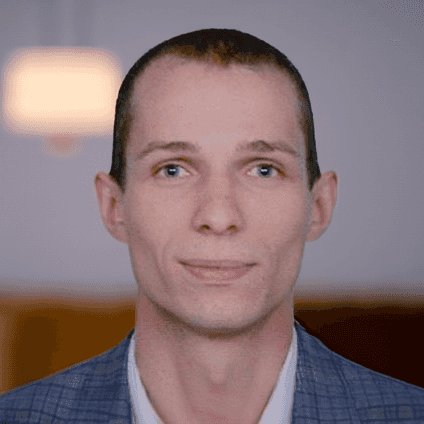
Head of AI/ML Center of Excellence
An experienced provider of financial solutions and artificial intelligence software development services, Itransition can build wealth management solutions featuring advanced analytics and automation capabilities to foster data-driven decision-making and maximize operational efficiency.
Table of contents
AI adoption trends in wealth management
of wealth management firms are in an advanced or intermediate stage of adopting AI-based automation
LSEG
of institutional investors think that AI and other disruptive technologies will improve their portfolio returns
PwC
the potential productivity gains for wealth managers from adopting GenAI in investment research
Oliver Wyman
Scheme title: Top areas by potential GenAI adoption impact according to wealth management firms
Data source: ey.com — The transformation imperative: generative AI in wealth and asset management
Scheme title: Assets under management of robo-advisors in US$ trillions
Data source: pwc.com — AI is transforming asset and wealth management
*Forecast
AI use cases in wealth management
Financial data analytics
Data analytics systems can process big data sets or streams of real-time information with AI and machine learning algorithms to derive insights and forecasts on key market trends, including stock price predictions, helping wealth managers make informed investment decisions. These solutions can facilitate the following financial analysis types:Â
- Fundamental analysis focusing on market and corporate indicators (market capitalization, dividend, etc.)
- Technical analysis to track asset price and trade volume trends over time and identify recurring patternsÂ
- Sentiment analysis to monitor the attitudes of investors about the market, typically shared on social media
Portfolio management
AI-enabled analyses and forecasts help wealth managers build investment portfolios of stocks, bonds, and other assets aligned with their clients’ long-term financial goals and risk tolerance. This process includes:
- Stock picking to identify stocks that are worth investing in and add them to a portfolio
- Asset allocation and diversification to spread investments across a suitable range of assets and lower risk
- Rebalancing to adjust an existing portfolio in line with evolving market conditions and the required risk-return profile
Robo-advisors
In an effort to expand their audience of retail investors beyond high-net-worth individuals, some financial institutions now offer automated financial planning and portfolio management via AI tools in addition to consultancy from human advisors. These digital advisors can:
- Ask clients about their financial goals and risk tolerance to create personalized financial plans
- Autonomously build and regularly rebalance clients’ portfolios based on personal and market data
- Direct users to a suitable human advisor whenever required
Customer relationship management
Artificial intelligence is making its way into the toolkit of marketing, sales, and service teams, powering finance CRM solutions that help streamline a wide range of client-related operations through:
- Automated lead segmentation to launch targeted marketing initiatives and foster new client acquisition
- Customer data consolidation to deliver financial advice tailored to client needs
- Automated customer support and personalized services via service bots to speed up case resolution and improve client experience
Middle- & back-office automation
By using AI technology, wealth management firms can automate time-consuming routine tasks and free up their staff to let them focus on client engagement and financial decision-making. Workflows that can easily be automated include:
- Financial data preparation and transformation to make it ready for analysis
- Generation and summarization of legal documents (contracts, invoices, etc.)
- Accounting operations like account balance cross-checking for bank reconciliation
Compliance management
Conventionally, wealth management firms rely on dedicated teams of professionals that ensure corporate compliance with everchanging standards and regulations. AI-based automation can help in this regard by:
- Extracting and summarizing investment compliance guidelines from IMAs, prospectuses, SAIs, and other documents
- Assisting with client profiling operations like KYC to prevent money laundering and other cases of fraud
- Taking care of routine cybersecurity tasks like password resets, account lockouts, and incident alerts
Enhance your wealth management operations with Itransition’s AI applications
Examples of AI tools for wealth management
MarketPsych sentiment analysis platform
MarketPsych is a financial analytics service provided by the London Stock Exchange Group. The platform relies on its own NLP engine to process market-relevant data from millions of news articles, social media posts, and other online sources in real time. Delving into this content, MarketPsych can monitor mentions and overall sentiment on companies, indices, stocks, commodities, and other entities or assets, along with more specific emotional indicators like optimism and uncertainty. This helps investment funds, banks, and other adopters predict market trends and optimize asset allocation.
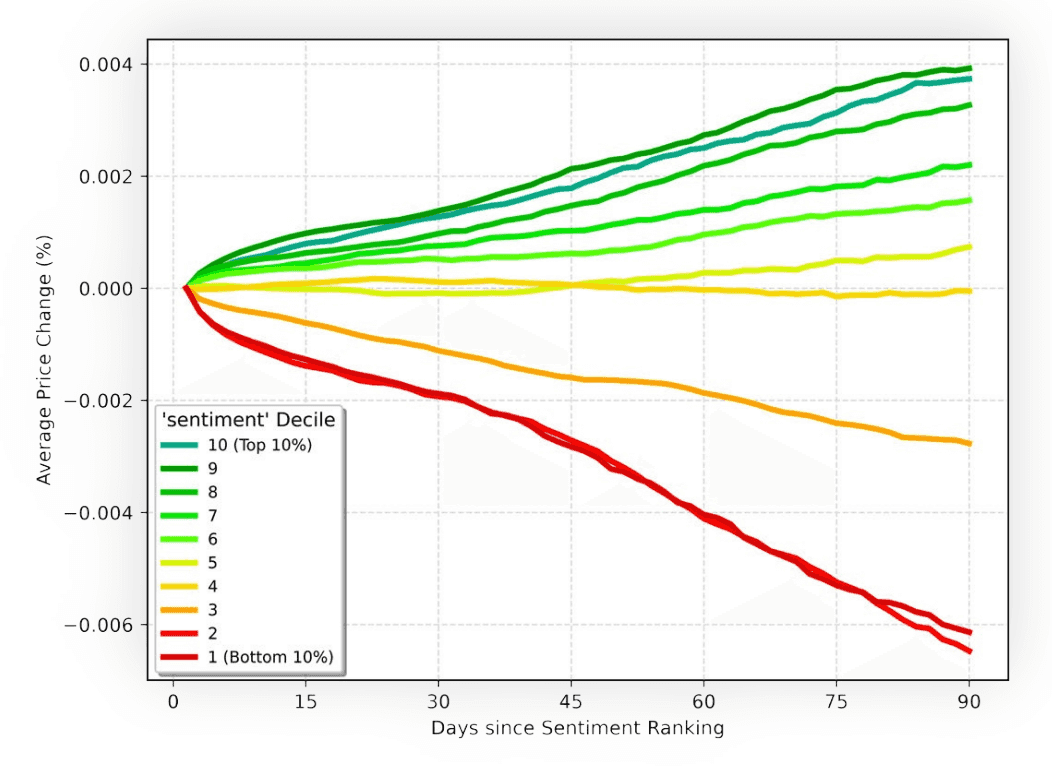
Image title: MarketPsych’s stock price forecasts based on market sentiment
Image source: lseg.com — MarketPsych Analytics from LSEG
Itransition developed a portfolio management platform for traders and investors featuring a custom machine learning algorithm to predict stock price trends based on historical and real-time market data. The solution helps users identify option trades with a good ROI/risk ratio and define an optimal stop price to enhance their trading strategies. Additionally, users can analyze portfolio risk based on each position’s volatility, redistribute risk between existing positions, and calculate the optimal investment size to create more balanced portfolios. The platform, whose algorithm has outperformed the S&P 500 index, serves thousands of users managing over $20 billion in investments.
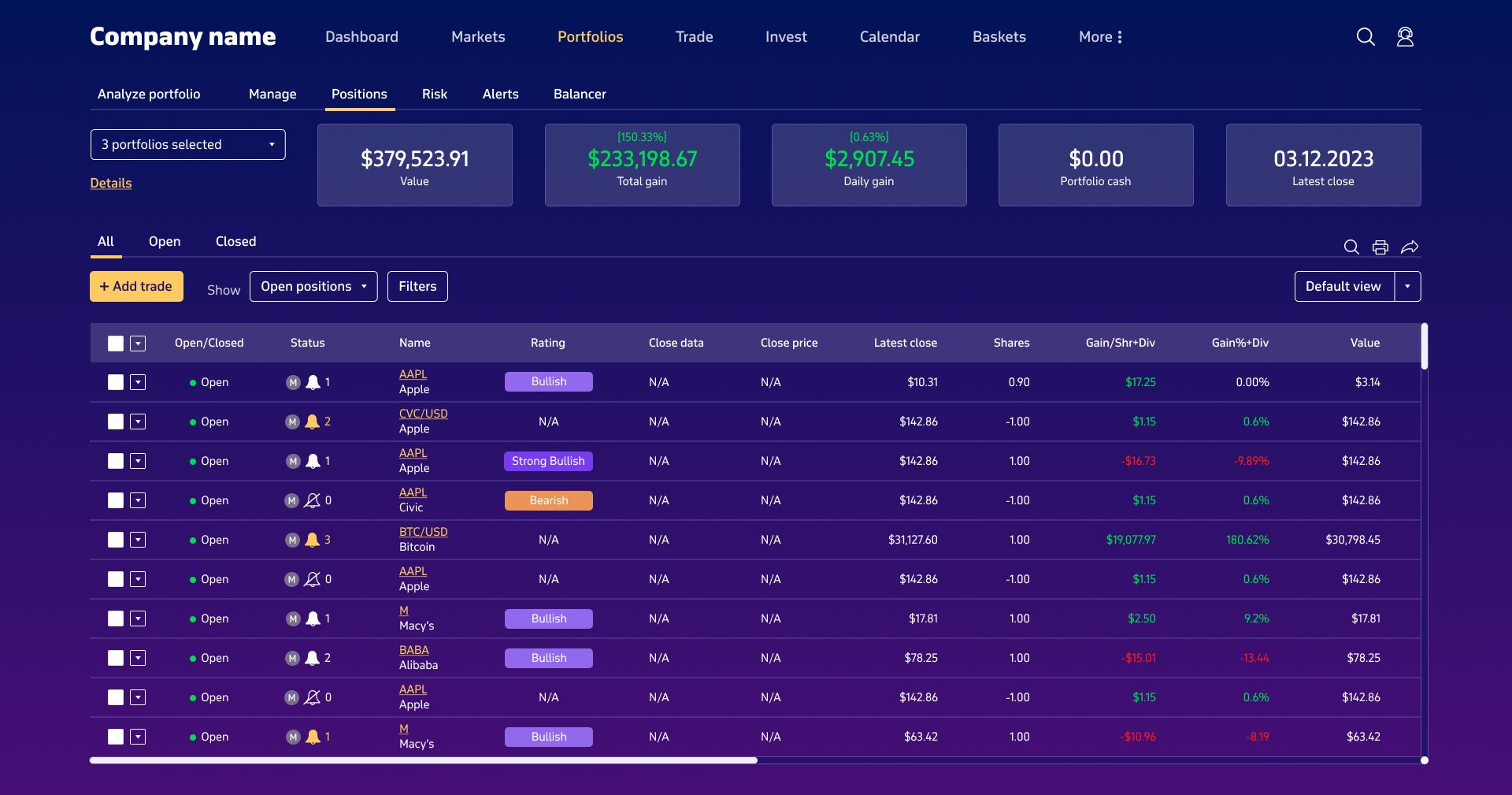
Image title: Portfolio positions dashboard
Image source: itransition.com — Dedicated team for investment portfolio management ecosystem
Vanguard’s robo-advisors
American investment management firm Vanguard enriched its service offering with three different robo-advisors (Digital Advisor, Personal Advisor, and Personal Advisor Select) addressing the needs of investors with different account sizes. These AI-based automated investment tools can assist users with risk tolerance assessment, financial planning, asset allocation and diversification, social security optimization, and tax-loss harvesting. That said, users are free to choose a hybrid plan combining automated wealth management and consultancy from human advisors. As of the end of 2023, Digital Advisor was the most popular robo-advisor in terms of assets under management ($289 billion).
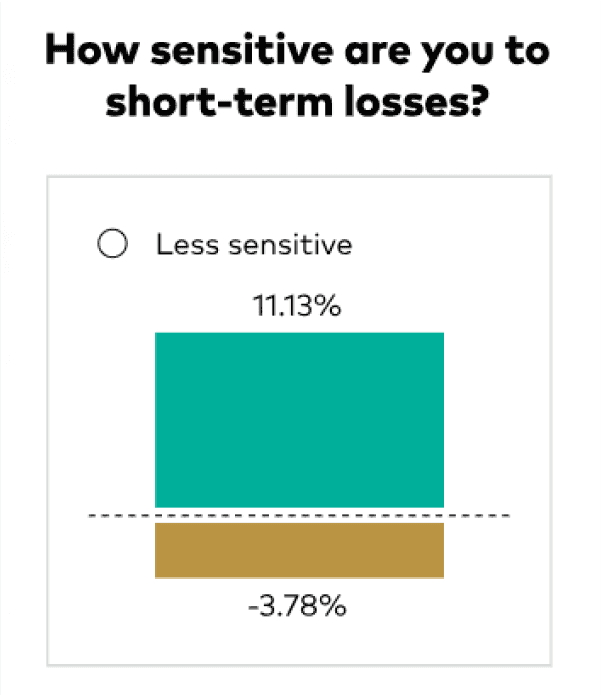
Image title: Vanguard’s Digital Advisor risk assessment feature
Image source: investor.vanguard.com — Automated investing with Digital Advisor
Financial Services Cloud is a cloud-based solution from the market-leading CRM provider Salesforce. This product complements generic Salesforce CRM functionality with industry-specific capabilities for various sectors of the BFSI macrogroup, including wealth and asset management. Adopters use advanced functionality powered by Einstein AI to improve their financial advisory services, such as customer analytics features to provide personalized account asset growth recommendations. This AI-enabled functionality will further expand in the upcoming months with new capabilities, including AI-generated client summaries providing financial advisors with details on customers’ financial status and goals.
Video title: Salesforce Financial Services Cloud CRM
Video source: salesforce.com — Unlock data to grow client relationships and AUM with trusted AI
Morgan Stanley Debrief note-taking chatbot
Morgan Stanley Wealth Management recently provided its financial advisors with a generative AI-powered chatbot to assist them with clerical tasks like note-taking to maximize their efficiency. This AI assistant can summarize Zoom meetings with clients (as long as they provide their consent) and generate email drafts covering key points discussed, allowing advisors to edit and send them at their discretion. Nearly all financial advisor teams have already adopted the tool, reporting time savings of about half an hour per meeting.
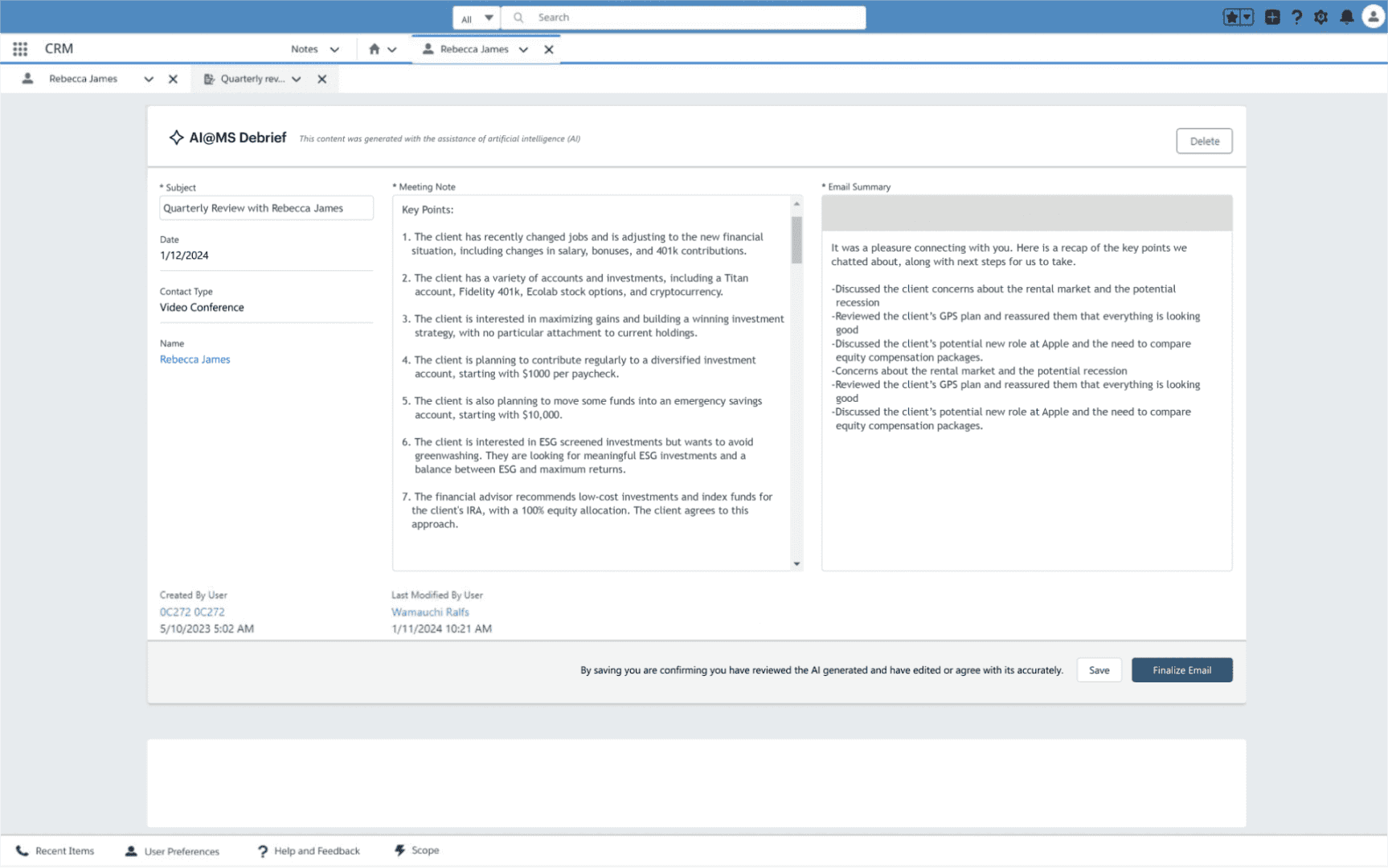
Image title: Morgan Stanley’s Debrief interface
Image source: cnbc.com — Morgan Stanley wealth advisors are about to get an OpenAI-powered assistant to do their grunt work
EY Sarge investment compliance automation solution
SARGE is a cloud-based AI tool for wealth and asset management firms developed by Ernst & Young and powered by machine learning and natural language processing algorithms. The solution can automatically extract investment guidelines from governing contracts and detect liabilities to facilitate compliance monitoring. EY estimates that adopting SARGE can reduce compliance management times by 75%.
AI adoption guidelines for wealth management firms
Data quality & availability
- Map available data sources to gather reliable, high-volume data sets for your analyses and derive accurate insights. These can range from market data providers like Nasdaq or S&P Global to government watch lists depending on the use case.
- Since financial data can be heterogeneous, set up ETL/ELT pipelines to integrate, transform, and store it into a suitable repository (such as a data warehouse to keep cleansed data ready for analysis or a data lake for large volumes of structured and unstructured data).
- Integrate your AI solution with selected data sources, including corporate systems and third-party services, to fuel it with an ongoing flow of information for analysis. You can enable seamless data exchange via API-based integrations or, alternatively, a middleware architecture like an ESB if data source systems use different communication protocols that must be converted.
- Consider leveraging cloud data integration services that offer ETL tools and pre-built integrations to facilitate the above tasks.
AI model training
- Depending on the task to perform, select suitable algorithms to process data and build the AI model that will power your wealth management solution (for instance, random forest for stock price prediction and K-Means clustering for customer segmentation).
- Cut down the set of input features considered by the model to the most relevant ones to speed up the training phase and make the resulting data model easier to interpret.Â
- Divide the financial data used to build your AI model into training, validation, and test sets. This helps mitigate overfitting, which can happen when a model is overtrained on specific data and ends up underperforming with other sets.
- If you lack the technology infrastructure to train an AI model, consider complementing your in-house processing resources with cloud-based services offering scalable computing power.
AI system reliability
- While no one can fully understand how AI models come to their conclusions (the infamous black box AI problem), you can use performance metrics like mean squared error to monitor model operation and identify potential reasons for bias and inaccuracies.
- The best-performing AI solutions are typically powered by deep learning algorithms like neural networks, which suffer from limited explainability due to their complex architectures. Wealth management firms should apply them carefully for selected tasks, avoiding their adoption in scenarios that require maximum transparency.
- AI model performance can degrade over time due to progressive changes in input data and related variables - a phenomenon known as model drift. You can define metrics to monitor model drift and, if detected, mitigate its effect by performing multiple retraining iterations with fresh data in line with MLOps' best practices.
Data privacy & security
- Train your AI model with obfuscated data sets to preserve the confidentiality of sensitive information. This implies anonymizing data through masking techniques like shuffling or substitution.
- Make sure your AI solution complies with data security regulations applicable to the wealth management industry, such as the GDPR and PCI-DSS. For instance, your software should incorporate features and techniques like identity and access management, data encryption, and multi-factor authentication to minimize cyber exposure.
- Adopt data governance policies and procedures to establish how data should be stored, accessed, and shared across your organization, including user roles and permissions for your wealth management software.Â
Itransition’s consultants share their expertise to help you streamline your AI project, overcome related challenges, and make the most of the resulting solution.
- Use case identification
- Current AI solution assessment
- Data mapping and quality audit
- Solution architecture design
- Tech stack selection
- Project roadmapping, budgeting, and ROI analysis
- Risk management strategy outline
- Development process supervisionÂ
- User training and support
Itransition develops AI solutions tailored to your unique requirements and industry specifics or modernizes existing software to keep up with emerging tech and business trends.
- ETL pipeline setup
- Data preprocessing (cleansing, transformation, etc.)
- Implementation of cybersecurity features
- AI algorithm selection and model training
- Front-end and back-end development
- Software integrations and APIs creation
- End-to-end testing
- Deployment to production
- Post-launch support, optimization, and upgrades
Looking for a reliable AI consulting and development partner?
Towards AI-powered wealth management
While artificial intelligence, including new technologies like GenAI, can be a valuable ally in automating time-consuming tasks and improving decision-making, wealth managers should implement it with due caution. First, the black-box nature of AI and complexity of its usage in certain scenarios suggest the need for constant human supervision. Second, this hybrid model combining AI output and human expertise is what investors actually want, at least for now. Research from LSEG shows that about 80% of investors are open to AI helping advisors manage their portfolios. However, less than half of them would let AI manage their portfolios directly.
An experienced IT partner like Itransition can help you address these and other challenges more effectively, building reliable and compliant AI solutions and facilitating their successful implementation in your day-to-day wealth management operations.
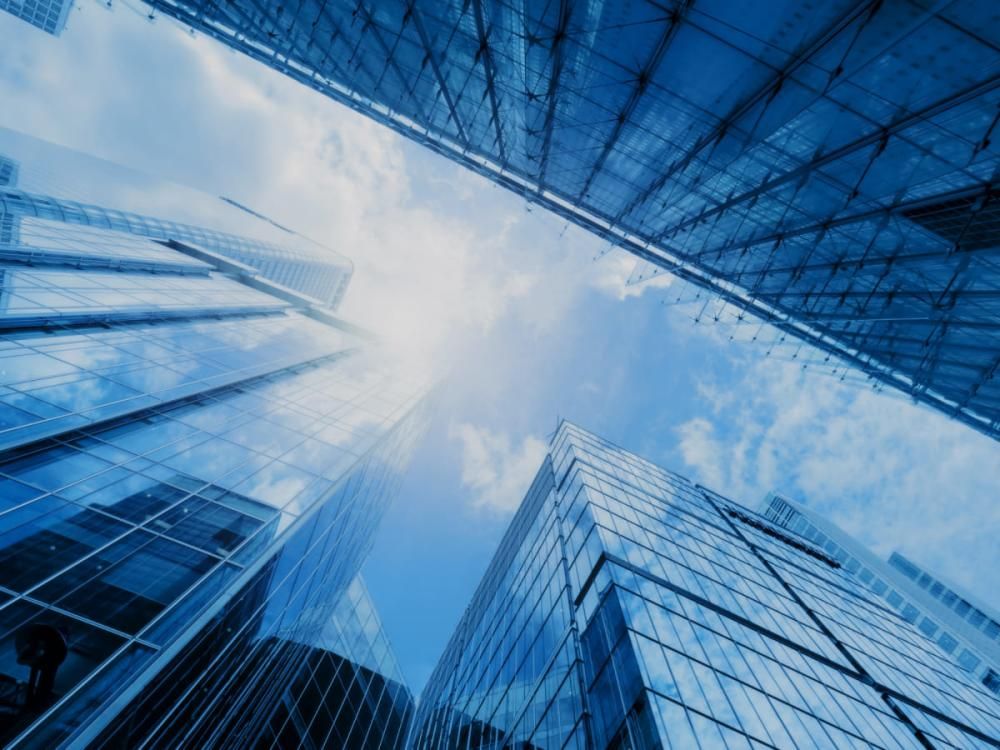
Insights
AI in fintech: use cases,
 solutions & implementation challenges
Explore the top use cases, real-life examples, and branches of AI in fintech, along with common adoption challenges and guidelines to address them.
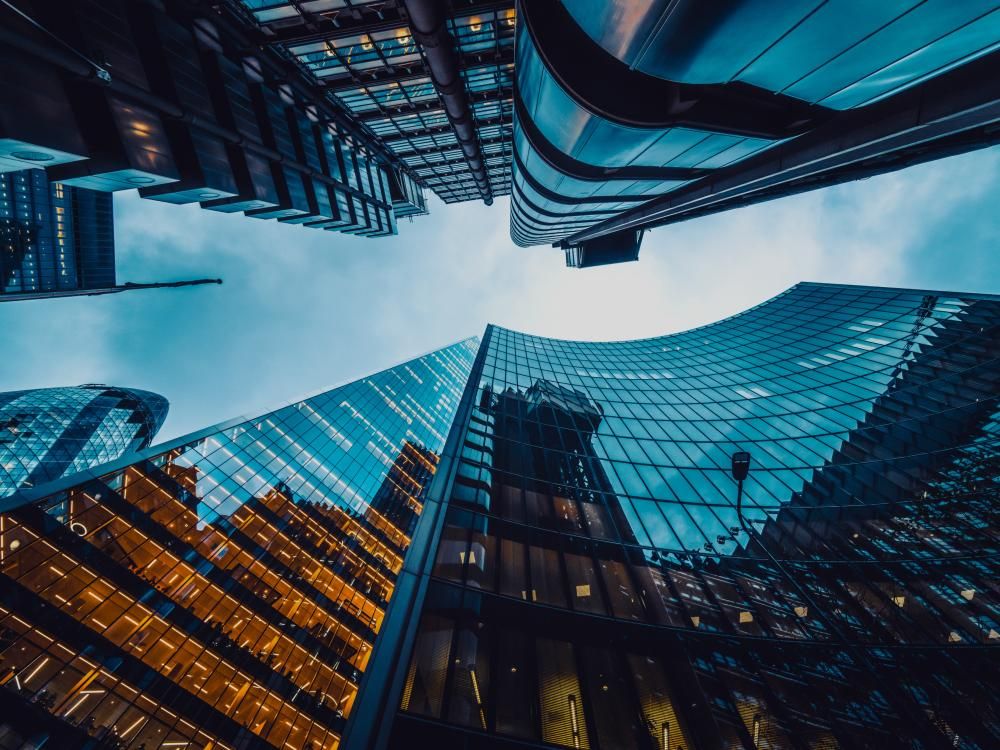
Insights
Predictive analytics in finance: use cases, platforms & adoption guidelines
Explore predictive analytics use cases, real-life examples, and models for the financial sector, along with top platforms and implementation best practices.
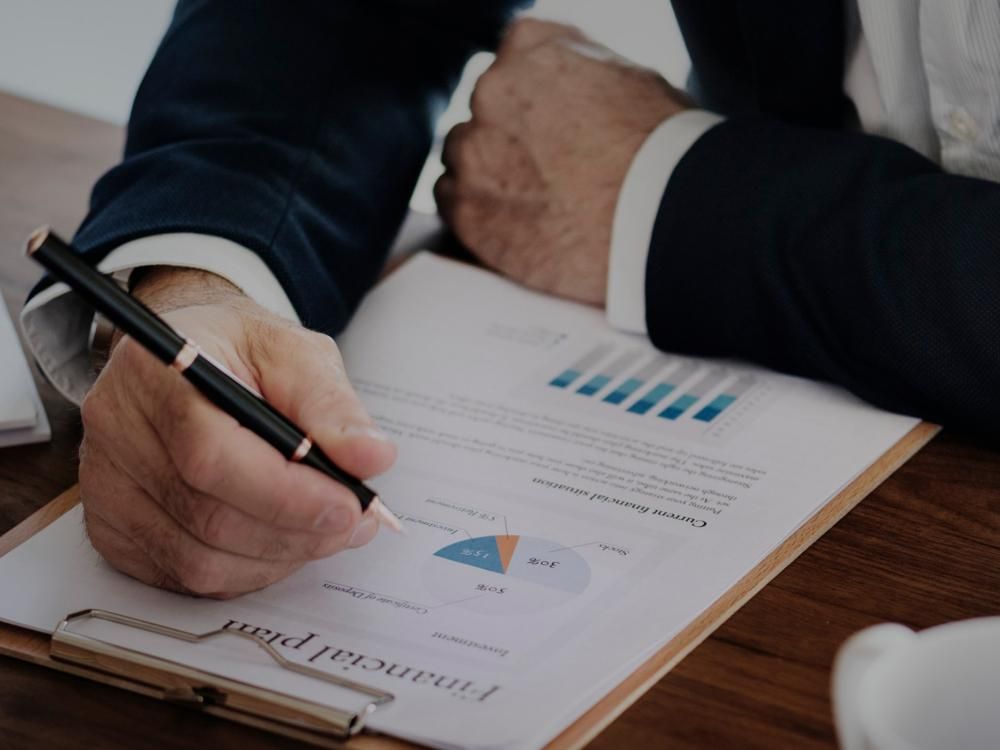
Case study
BI consulting and engineering for a commercial bank
Find out about Itransition's high-profile BI consulting for a Canadian bank, including data architecture analysis and a BI strategy.
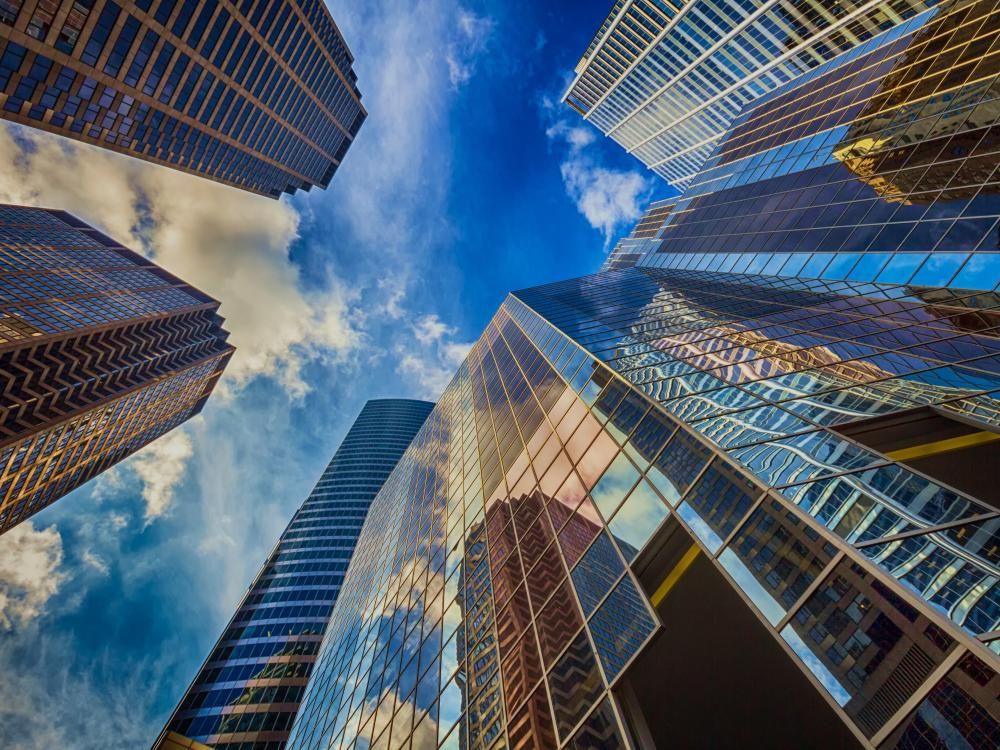
Insights
Business intelligence for finance: capabilities, tools & integrations
Explore how business intelligence and data analytics benefit the financial services sector and learn what capabilities, integrations, and tools make up a successful BI strategy.
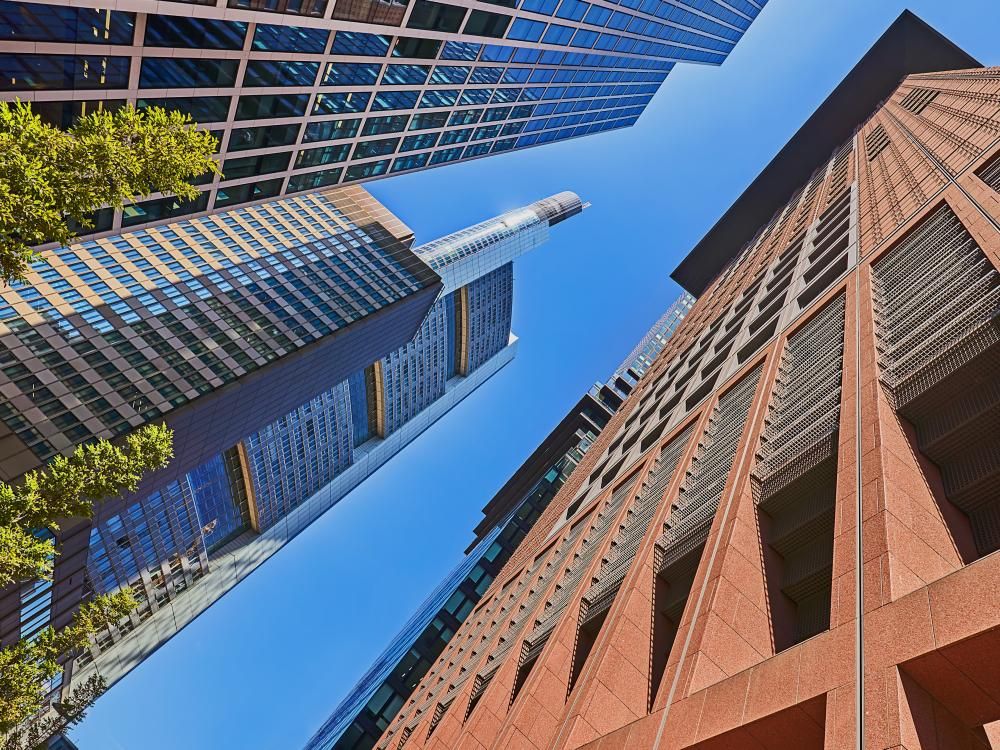
Insights
Machine learning in banking: 8 use cases and implementation guidelines
Learn how banks can apply machine learning to improve customer engagement, streamline compliance, and drive more revenue.
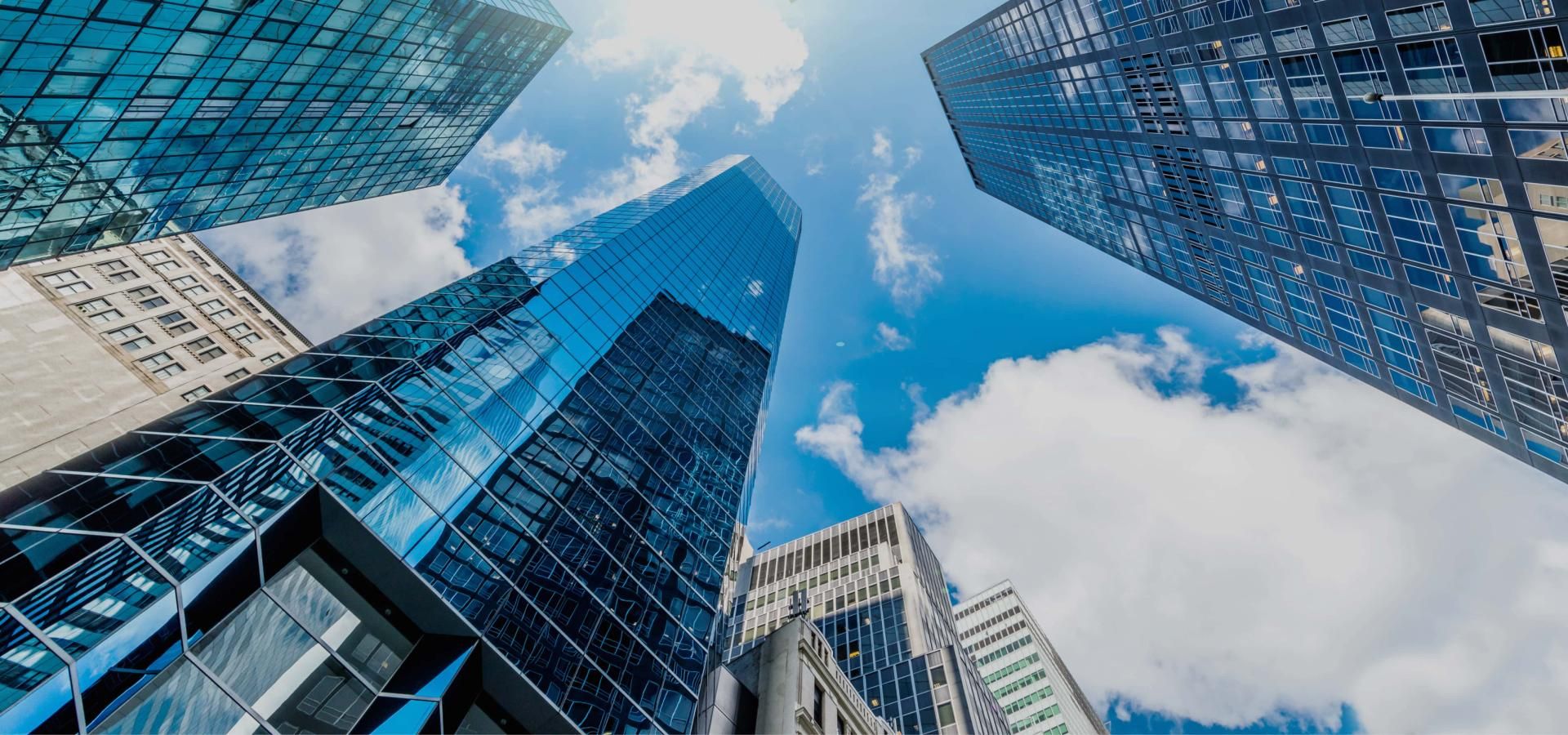
Service
Stock trading software development services
Explore Itransition’s stock trading software development services, along with solution types, features, and AI and blockchain capabilities we deliver.